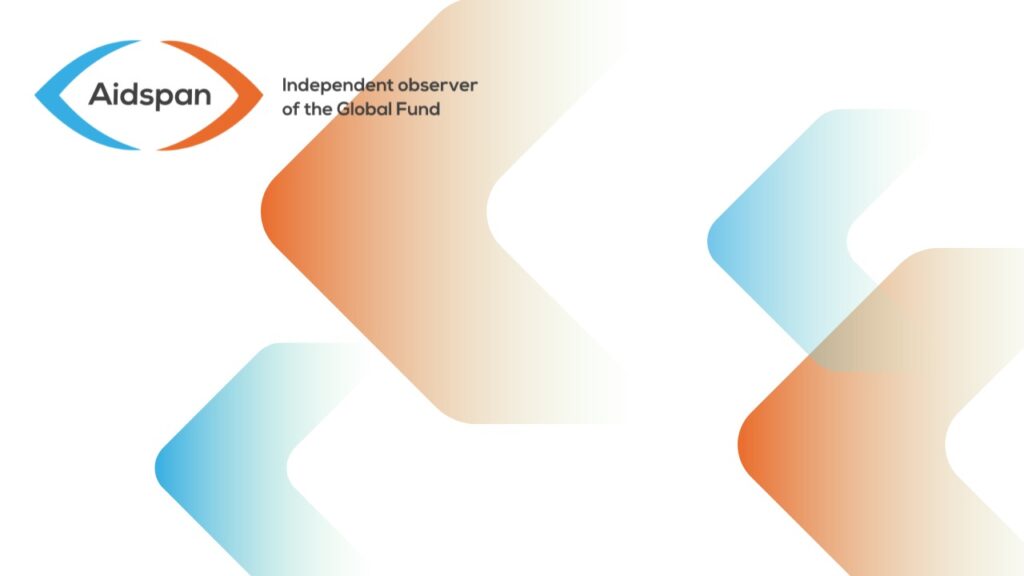
INCOMPLETE AND INACCURATE DATA REPORTED IN 11 COUNTRIES FOR 2019-2020
Author:
Download PDF As countries finalize their funding requests to the Global Fund or negotiate their grants for the 2020-2022 allocation cycle, it becomes important to reflect on systemic issues reported in the previous allocation cycle. Our review of the Office of the Inspector General (OIG) audit reports for 2019-2020 reveals that inaccurate and incomplete data is the third leading issue…
Article Type:Article Number:
A review of the audit findings of the Office of the Inspector General
ABSTRACT Our review of the Office of the Inspector Generalās audit revealed that incomplete data and data inaccuracies were a systemic problem in some countries during 2019 and 2020. It demonstrates health system weaknesses that all stakeholders need to address. There is a need for increased funding for resilient and sustainable systems for health to support countries to manage their data systems better. Specifically, the additional funding should be used to train healthcare workers in data collection and inculcate a culture of data analysis for decision making.
As countries finalize their funding requests to the Global Fund or negotiate their grants for the 2020-2022 allocation cycle, it becomes important to reflect on systemic issues reported in the previous allocation cycle. Our review of the Office of the Inspector General (OIG) audit reports for 2019-2020 reveals that inaccurate and incomplete data is the third leading issue plaguing Global Fund grants.
In the previousĀ GFO (Issue 387), published on 1 October 2020, we highlighted gaps in oversight and assurance arrangements, and challenges in the design and implementation of interventions as the leading audit findings.
Information for this article comes from the Global Fundās audit of the grants for the years 2019 and 2020, and other publicly available documents.
Countries with data audit
We focused on 11 countries where the Global Fund found that the collection and recording of accurate data was a challenge. All these countries are in Africa, except Papua New Guinea in Oceania (Table 1). Seven of these countries have a larger Global Fund portfolio, higher disease burden, and are higher risk and thus classified as ācoreā, while the remaining four have a very large Global Fund portfolio and mission-critical disease burden, thus classified as āhigh impactā.
Eight of these countries are under Additional Safeguard Policy (ASP), which involves instituting additional measures to protect Global Fund investments. Three of these countries have an extremely high external risk index due to weak governance and human-made or natural disasters and are therefore classified as Challenging Operating Environments (COEs). In six of these countries, the Principal Recipients (PRs) were state agencies, such as government ministries and corporations.
Table 1: Characteristics of countries with data challenges
No | Country | Region | Category | ASP | COE | PR |
1 | Angola | Africa | Core | No | No | Both state and non-state PRs |
2 | Benin | Africa | Core | No | No | State PR |
3 | DRC | Africa | High Impact | Yes | Yes | Both state and non-state PRs |
4 | Ghana | Africa | High Impact | No | No | State PR |
5 | Malawi | Africa | High Impact | No | No | State PR |
6 | Papua New Guinea | Oceania | Core | Yes | No | Non-state PR |
7 | Rwanda | Africa | Core | No | No | State PR |
8 | Sierra Leone | Africa | Core | No | Yes | State PR |
9 | South Sudan | Africa | Core | Yes | Yes | Non-state PR |
10 | Togo | Africa | Core | No | No | State PR |
11 | Zimbabwe | Africa | High Impact | Yes | No | Both state and non-state PRs |
Source: Aidspan, based on the OIG audit reports for 2019-2020
Note: DRC ā the Democratic Republic of Congo; State PRs are government ministries or agencies while non-state PRs are non-governmental organizations
Data-related challenges reported in these countries fall under two main categories: data discrepancies and incomplete data.
Data discrepancies
Data discrepancies were widespread in disease programs across countries. Unreliable data makes it hard to assess the performance and impact of interventions.
InĀ Angola, the HIV, tuberculosis (TB), and malaria indicators reported in the central registry and in the facilities varied by over 27% in more than 75% of the health facilities that the audit team visited. Also, data on the number of people living with HIV on antiretroviral treatment (ART) and community-based malaria interventions were unreliable. Similar discrepancies between the reported and actual number of people living with HIV on ART existed inĀ the Democratic Republic of Congo, with at least 10% over-reporting in five of the 13 health facilities sampled. In Malawi, the OIG found that the number of malaria cases suspected, treated, and reported was overstated by 13%, 29%, and 28%, respectively.
InĀ Rwanda, there were differences in drug consumption and patient data, i.e., the pharmacy data and the consultation data were not similar. InĀ Sierra Leone, the OIG noted erroneous data entry. InĀ Togo, the OIG noted inaccurate HIV and malaria data since 42% of reported results for pregnant women on ART under the Prevention of Mother to Child Transmission program could not be reconciled with patient records, while the malaria indicators had discrepancies greater than 10%. InĀ Zimbabwe, the OIG identified HIV data anomalies since the program data was higher than the estimate: the number of people living with HIV was underestimated.
InĀ Papua New Guinea, the OIG questioned the accuracy of TB records. The country relies on estimates obtained from the World Health Organization (WHO) in the absence of a national TB survey. According to the OIG, there was a need to assess the accuracy of the TB burden recorded.
Incomplete data
InĀ Ghana, the OIG said that the data reported for HIV and TB was incomplete. Two of the four teaching hospitals, 45 of the 375 public ART centers (12%), and some private health facilities do not enter data in the District Health Information System (DHMIS2). Similarly, in Sierra Leone, a third of health facilities sampled reported fewer than a quarter of HIV and malaria indicators. Private and district facilities, which account for 21% of facilities offering HIV, TB, and malaria services, were not included in the national health information system. Also, the OIG noted that there were stockouts of data reporting tools for over a week in half of the health facilities reviewed.
Some immediate causes of data inaccuracies: inadequate controls and assurance over data
An immediate cause of inaccurate data was the limited data quality assurance mechanisms in many countries. For example, in Rwanda, the data systems were not secured in the system as health facilities could change the reported malaria data without being required to seek approval. About two-thirds (63%) of community health workers lacked physical registers to support the data that they entered for three months. In Sierra Leone, the OIG noted that there were also stockouts of data reporting tools for over a week in half of the health facilities reviewed.
InĀ South Sudan, the OIG noted that inadequate funding, understaffing, delayed strengthening of the national health information system and a lack of key national surveys contributed to the data issues for the three diseases.
Some systemic factors contribute to data-related challenges
It is important to place these data issues in context: most of these countries are low income and fragile. The low level of income per capita is one of the reasons that they are eligible for the Global Fund grants; the burden of the disease is the second reason.
Various donors with specific and different data collection, and reporting systems
Support for these countriesā health sectors is provided by several bilateral or multilateral institutions, which often come with their specific data collection and reporting requirements. AĀ review of donor reporting requirementsĀ by the United Nations in 2017 indicated that these are outlined in the signed donor agreements and vary significantly in terms of the format, detail, and frequency. With different systems for different diseases and conditions, the data often becomes fragmented. This disjointed system greatly reduces the possibility of obtaining reliable data for different conditions in the same country.
Healthcare workers training and motivation
The inadequate number of human resources in health facilities contributes to the poor quality data because their priority, often rightly so, is to provide adequate care.
The attitude, confidence, and competence of health workers in performing data collection play a significant role in determining the quality and accuracy of health data. AĀ study conducted in BeninĀ in 2012 revealed that carelessness, limited knowledge on the usefulness of data, a lack of motivation, and the competence of staff influenced the quality of health data.
When health workers fail to recognize the importance of data collection and consider it a waste of time, it hinders the collection of accurate and reliable data. However, when healthcare workers acknowledge that data is useful for improving services, and not merely to meet district or program targets, theĀ quality of the data collected and the services provided can improve.
It is often debated whether healthcare workers should be given additional motivation to collect data and conduct analysis. Some argue that financial motivation is necessary to make them pay serious attention to the quality of data collected, especially during busy clinic days. Critics contend that the motivation may induce the falsification of data, especially in a performance-based financing system where the payment depends on the number of patients attended to.
Paper and electronic systems co-exist
Some countries use a hybrid system of data collection with both paper-based and electronic systems. For example, the staff at the facility level uses tablets to capture and then transmit data electronically to the district level. Paper-based data systems are laborious as it involves capturing data on paper and then entering the data into a database. The transposition of data is prone to errors and is time-consuming.
Other causes
There are other causes of poor data quality. The OIG noted that the collection of accurate health data is a low priority. Irregular support supervision, limited coordination, and accountability for community-level data were added factors that contributed to inaccuracies in program data.
Resilient and sustainable systems for health grants can help cure data challenges
The Global Fund provides performance-based grants. Thus, reliable data underpins its work. Accurate, timely, and reliable data is needed to measure program progress. Data inaccuracies reported in 2019-2020 demonstrate health system weaknesses that all stakeholders need to address. The Global Fund can support countries to achieve improved data accuracy through additional funding for resilient and sustainable systems for health (RSSH). The additional funding should be directed towards strengthening national health information systems and training healthcare workers on the importance of capturing accurate data. The training should specifically aim to change the attitudes and behaviors of healthcare workers and inculcate a culture of data collection and analysis for decision making at the health facility level.
Further reading
- Audit reports for the 2019-2020Ā period can be accessed at the Global Fund’s Audits & Investigations webpage